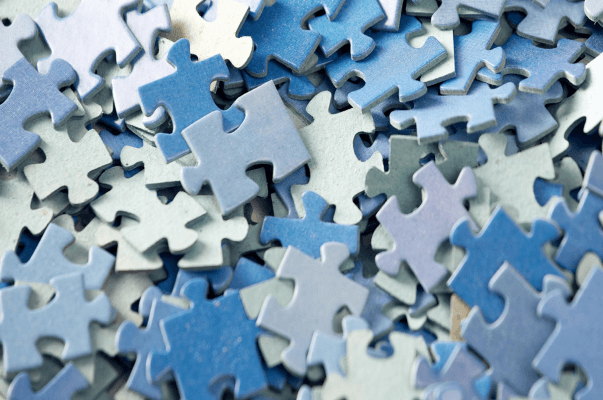
The Generative AI Revolution: 9 Trends Reshaping Healthcare in 2025
Date
Tue, Feb 4, 2025, 06:00 AM
Become a CHIME25 Ambassador: bring your team at a discount and unlock VIP perks Apply Today!
Date
Tue, Feb 4, 2025, 06:00 AM
January 2025
In November 2022, OpenAI launched ChatGPT, which became the fastest-growing consumer application of all time within one month. In the intervening two years, massive investment in Generative AI across industries has created unprecedented potential for healthcare transformation.
Despite this scale of investment and potential, Generative AI (Gen AI) has not yet transformed healthcare. While Gen AI infrastructure is more accessible than ever and clinicians are adopting it faster than previous AI technologies, most hospital systems remain in an experimental phase, focusing on disparate use cases and point applications that have yielded only peripheral productivity gains.
As we move into 2025, we anticipate a pivotal shift: Gen AI will usher in the era of the AI-Powered Clinician of the future. This transformation will redefine the talent landscape, with the primary constraint moving from technical roles to knowledge workers and their ability to embrace these new technologies. In this article, we examine both the current state of healthcare AI adoption and the transformative trends we expect to unfold over the next 12 to 24 months.
Generative AI is hailed as a game-changer across industries, with investments soaring from $1.7 billion in 2022 to $14 billion in 2023, and projected to reach $109 billion by 20301. Most of the funding is funneled into AI assistants, Human-Machine Interfaces (HMI), and generative tools. Over half of Generative AI startups are in early-stage funding, illustrating the nascency of most Generative AI applications. These immature tools provide narrow point solutions and fall short of reimagining how processes are executed and jobs are reconfigured.
Hospitals also face outdated infrastructure with limited cloud strategies, fragmented workflows, and a cautious approach to change—barriers that hinder the smooth incorporation of Gen AI. Forward-thinking leaders are advocating for responsible data sharing and aligning AI initiatives with organizational goals to move beyond pilot projects.
The Gen AI ecosystem is crowded and teems with opportunity, attracting startups, hyperscalers,
and health incumbents. Startups often struggle to scale past regulatory hurdles and compete with hyperscalers like Microsoft, Amazon, Meta, and Google, which aggressively pursue hospital partnerships despite skepticism over data privacy risks and ROI. Meanwhile, health tech leading incumbents, particularly those offering EHR-focused solutions, integrate AI cautiously, making incremental improvements as their legacy architectures often constrain them. These efforts typically extend existing functionalities, such as enhancing patient communication and engagement tools, rather than reimagining workflows or care delivery models. In fact, our analysis of major EHR incumbents’ AI plans suggests there is significant whitespace (50 – 90% across 8 key domains (e.g. clinical operations, patient experience etc.) in terms of innovation opportunity and value creation potential
Since the launch of ChatGPT, organizations have embraced Generative AI primarily to enhance productivity, with 92% of users leveraging it for this purpose and 43% reporting the highest ROI in this area, according to IDC’s 2024 AI Opportunity Study 2. In healthcare, AI adoption is advancing beyond productivity gains to tackle complex challenges such as reducing claim denials and improving payer communications. This evolution marks a pivotal shift, but many health systems remain caught between experimentation and enabling the changes via at-scale transformation.
Thus far, hospitals have rapidly acquired and deployed AI solutions, building expansive portfolios of new functionalities focused on individual use cases. While these efforts have yielded some successes, they’ve also exposed significant struggles. AI solutions are technically complex and costly requiring intensive training and collaboration between technical and business teams.
Source: Impact Advisors
Read full article by downloading the PDF below.